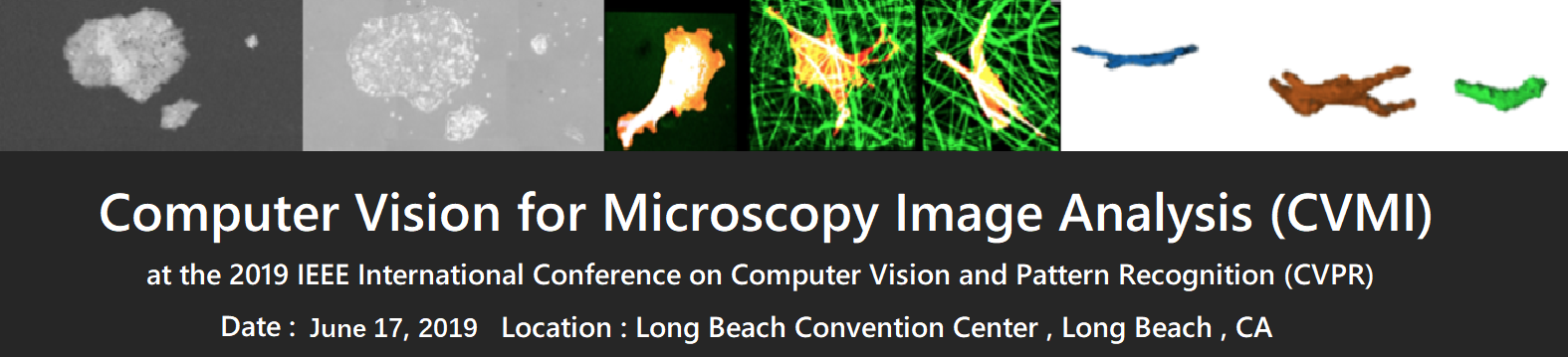
Invited Speakers
Title: The ImageJ Ecosystem: An Open and Extensible Platform for Biomedical Image Analysis
Start Time: 9:00 AM
Speaker: Professor Kevin Eliceiri, Director, Laboratory for Optical and Computational Instrumentation, University of Wisconsin–Madison
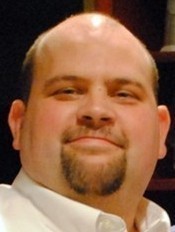
Abstract: Biological imaging has greatly advanced over the last thirty years with the now unprecedented ability to track biological phenomena in high resolution in physiologically relevant conditions over time and in space. As these imaging technologies mature and become main stream tools for the bench biologist there is great need for improved software tools that drive the informatics workflow of the imaging process from acquisition and image analysis to visualization and dissemination. To best meet the workflow challenges, these tools need to be freely available, open source, and transparent in their development and deployment. In particular it is clear that given the complexity, and heterogeneity of the modern image dataset, there cannot be a single software solution. Different imaging processing and visualization approaches need access not only to the data but also to each other. There needs to be compatibility not only in file import and export but interoperability in preserving and communicating what was done to the image. There is a great opportunity in achieving this interoperability, tools that can talk to each other not only enable new biological discovery but also efficiencies in sharing code and in many cases more precise workflows. We present our efforts towards interoperability and extensibility in the ImageJ consortium including partners such as the Cell Profiler, FIJI, KNIME, and Open Microscopy Environment groups. We are actively developing key software libraries like Bio-Formats, ImgLib and ImageJ Ops that are utilized to analyze and visualize biological image data, to the developmental benefit of not only of the applications but the libraries themselves.
Bio: Dr. Kevin Eliceiri is the Walter H. Helmerich Research Chair and Associate Professor of Medical Physics and Biomedical Engineering at the University of Wisconsin at Madison. He is an Investigator in the Morgridge Institute for Research and member of the Carbone Cancer Center and McPherson Eye Research Institute. He is director of the Laboratory for Optical and Computational Instrumentation, a biophotonics laboratory dedicated to the development and application of optical and computational technologies for cell studies. The Eliceiri lab is a lead developer in several open source imaging packages including FIJI and ImageJ. His instrumentation efforts involve novel forms of polarization, laser scanning and multiscale imaging. Dr. Eliceiri has authored more than 180 scientific papers on various aspects of optical imaging, image analysis, cancer and live cell imaging.
Title: Phenotypic Screening of HiPSC Derived Neurons: Balancing Throughput with Relevance
Start Time: 9:30 AM
Speaker: Dr. Anne Bang, Director, Cell Biology, Conrad Prebys Center for Chemical Genomics at Sanford Burnham Medical Research Institute
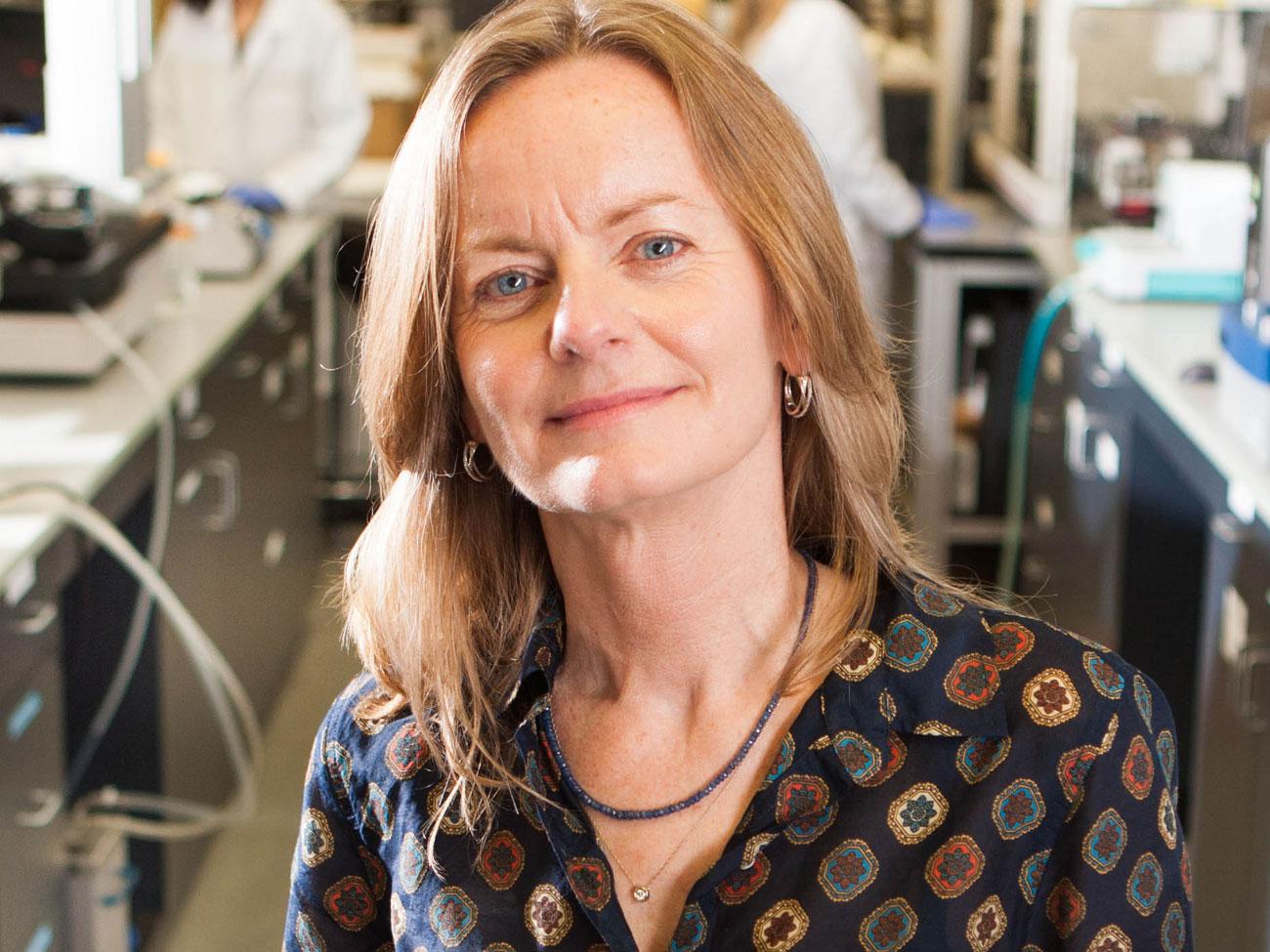
Abstract: Patient specific induced pluripotent stem cells (iPSC) complement traditional cell-based assays used in drug discovery and could aid in the development of clinically useful compounds. They are scalable, allow interrogation of differentiated features of human cells not reflected by immortalized lines, and importantly, carry disease-specific traits in complex genetic backgrounds that can impact disease phenotypes. Development of technology platforms to perform compound screens of iPSC with relatively high-throughput will be essential to realize their potential for disease modeling and drug discovery. Towards this goal, we have been working to develop assay platforms to interrogate fundamental aspects of neuronal morphology and physiology, providing a basis for further development of more complex phenotypic readouts and compound screens based on patient specific hiPSC-derived neurons. We will discuss our screening results and development of patient cell specific and hiPSC based models for testing of drugs on disease relevant cell types.
Bio: Dr. Anne Bang joined the Sanford Burnham Prebys Medical Discovery Institute in June 2010 as Director of Cell Biology at the Conrad Prebys Center for Chemical Genomics, a state-of-the-art academic drug discovery center. Her current research efforts are directed at developing patient-specific, induced pluripotent stem cell (iPSC)-based disease models of neurological disease that reflect higher order cellular functions and recapitulate disease phenotypes yet have the throughput and robustness necessary for drug discovery. Her goal is to use these models for target ID and drug screening to develop clinically useful compounds. Prior to joining SBP she served as Director of Stem Cell Research at ViaCyte Inc, where her efforts focused on process optimization and advancing Viacyte’s cell therapy product into development, scaled manufacturing, product characterization, and safety assessment. Dr. Bang received a B.S. from Stanford University, a Ph.D. in Biology from UCSD, and was a post-doctoral fellow at the Salk Institute.
Title: Case Study on Applying Deep Learning Methods to High Content Image-Based Assays
Start Time: 10:00 AM
Speaker: Subhashini Venugopalan, Google Accelerated Science Team, Google Research
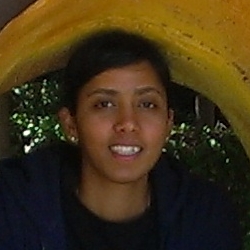
Bio: Subhashini Venugopalan is a research scientist with Google applying machine learning to medical images and audio. She received her PhD from University of Texas at Austin working on problems in the intersection of computer vision, deep learning, and natural language processing. She was a recipient of the University of Texas Dissertation Fellowship. As a reviewer, she has served on the program committee of several conferences (CVPR, AAAI, NAACL, ACL, EMNLP) and journals (IJCV, TPAMI), and has received an outstanding reviewer award (EMNLP 2018). Subhashini received her master's degree from Indian Institute of Technology (IIT) Madras, and a bachelor's degree from National Institute of Technology (NIT) Karnataka, India.
Title: What Concussions Do to Brain Cells: A Deep Look
Start Time: 13:00 PM
Speaker: Professor Badri Roysam, Chair of Electrical and Computer Engineering, University of Houston
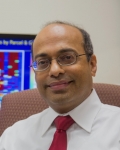
Abstract: At the cellular level, traumatic brain injury (TBI) initiates a complex web of pathological alterations in all the types of brain cells, ranging from individual cells to multi-cellular functional units at multiple scales ranging from niches to the layered brain cytoarchitecture. Unfortunately, current immunohistochemistry (IHC) methods reveal only a fraction of these alterations at a time, miss the many other alterations and side effects that are occurring concurrently, and do not provide quantitative readouts. The potential consequence of unobserved and untreated cellular alterations is high, as they may contribute to confounding, co-morbid, or persistent conditions (e.g., depression, headaches, stress-related health problems). Importantly, the current state of drug development for brain pathologies leaves much to be desired, with a recent review concluding that “most of the pharmacologic and non-pharmacologic treatments have failed to demonstrate significant efficacy on both the clinical symptoms as well as the pathophysiologic cascade responsible for the permanent brain injury”. In this talk, I will describe a practical approach to pathological brain tissue mapping with a focus on combination drug treatment. Our approach is based on replacing the many low information content assays with a single comprehensive assay based on imaging and analyzing highly multiplexed whole brain sections using 10 – 50 molecular markers, sufficient to analyze all the major brain cell types and their functional states over extended regions.
Bio: Badri Roysam (Fellow IEEE, AIMBE) is the Hugh Roy and Lillie Cranz Cullen University Professor, and Chairman of the Electrical and Computer Engineering Department at the University of Houston (2010 – present). From 1989 to 2010, he was a Professor at Rensselaer Polytechnic Institute in Troy, New York, USA, where he directed the Rensselaer unit of the NSF Engineering Research (ERC) Center for Subsurface Sensing and Imaging Systems (CenSSIS ERC), and co-directed the Rensselaer Center for Open Source Software (RCOS) that was funded by a major alumnus gift. He received the Doctor of Science degree from Washington University, St. Louis, USA, in 1989. Earlier, he received his Bachelor’s degree in Electronics from the Indian Institute of Technology, Madras, India in 1984. Badri’s research is on the applications of multi-dimensional signal processing, machine learning, big-data bioinformatics, high-performance computing to problems in fundamental and clinical biomedicine. He collaborates with a diverse group of biologists, physicians, and imaging researchers. His work focuses on automated analysis of 2D/3D/4D/5D microscopy images from diverse applications including cancer immunotherapy, traumatic brain injury, retinal diseases, neural implants, learning and memory impairments, binge alcohol, tumor mapping, stem-cell biology, stroke research, and neurodegenerative diseases.
Title: Accelerating Drug Discovery Through the Power of Microscopy Images
Start Time: 13:30 PM
Speaker: Allen Goodman, senior software engineer at Broad institute of MIT and Harvard
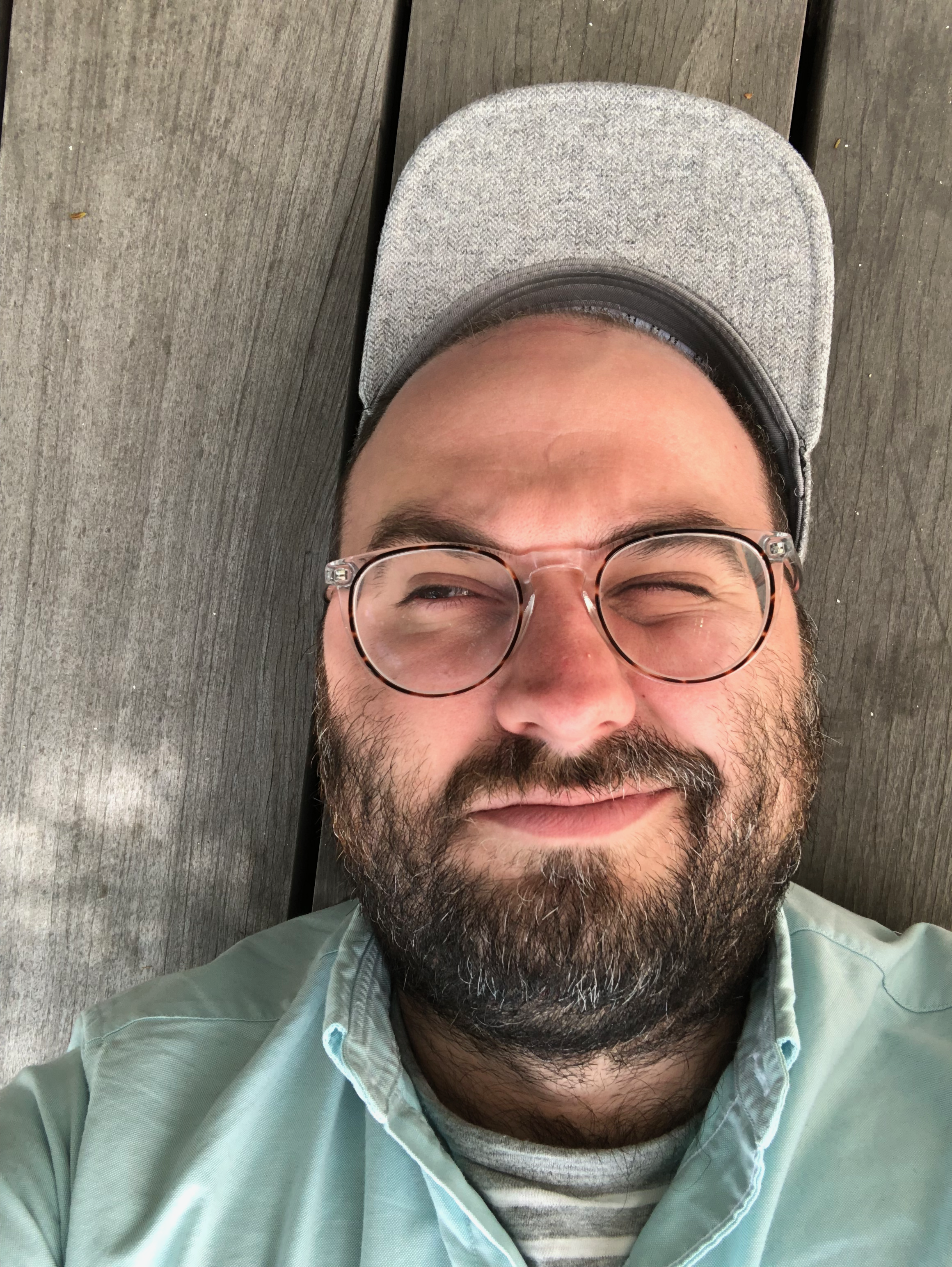
Abstract: An overview of a user-friendly deep learning-based application in collaboration with the Horvath laboratory. This browser-based phenotype classifier uses deep learning and is yet to be named. It will replace the Carpenter lab's prior CellProfiler Analyst and the Horvath lab's Advanced Cell Classifier tools and is currently in prototype stage.
Bio: Allen Goodman is a gifted software engineer, recognized for writing pioneering web and mobile applications. He was a founding engineer and Director of Research of Simple, a highly successful customer-oriented online bank startup, where he co-wrote their critically acclaimed mobile and web applications. Then, as a senior software engineer for Chef—the leading software automation company—he co-wrote Chef Analytics, a real-time message broker for cloud computing. His technical expertise includes cross-platform proficiency, fluency in general-purpose and scientific programming languages, and expert knowledge of popular tools, methodologies, and best practices. Goodman decided to apply his software engineering talents to biomedicine and joined the Carpenter laboratory in August 2015, where he has dramatically reshaped CellProfiler, and is leading the group’s efforts in developing new bioimage analysis tools based on deep learning methods. He was also recently named an Imaging Software Fellow, an award by the Chan Zuckerberg Initiative to support funding of open-source software efforts to improve image analysis and visualization in biomedicine.
Title: Interoperable Web Computational Plugins for Large Microscopy Image Analyses
Start Time: 16:20 PM
Speaker: Peter Bajcsy, NIST and Nathan Hotaling, NIH
![]() |
![]() |
To address the above challenges, groups have developed solutions that leverage modern web technologies on the client side and a spectrum of databases, computational workflow engines, and communication protocols on the server side to hide the infrastructure complexity. However, these solutions have not focused on inter-operability, specifically as it relates to domain specific computational plugins.
To address the inter-operability of computational web plugins and to develop an open source platform for executing web-based image processing pipelines over very large image collections, the National Institute of Standards and Technology (NIST) along with the National Institutes of Health (NIH) - National Center for Advancing Translational Science (NCATS) have formed a close collaboration. The plugins developed by both institutes are based on software containers as standardized units for deployment, as well as on dynamically created web user interfaces (UI) to enter parameters needed for the software execution. Each container packages code, with all its dependencies, and has an entry point for running the computation in any computing environment. Each UI description file contains metadata about the plugin container and the computation parameters.
We will demonstrate the utility of the pipeline system with web plugins by analyzing large multi-channel fluorescent images of whole murine eyes to assess increased accumulation of auto- fluorescent waste products in c57bl/6 mice with gene ABCA4 selectively knocked-out (KO) to quantitatively assess disease state and progression in both KO and control models. With the NIST and NIH NCATS combined efforts, researchers are enabled to discover quantitative insights from their imaging data and reuse computational tools developed by anyone following the web computational plugin conventions.
Bio: Dr. Peter Bajcsy received his Ph.D. in Electrical and Computer Engineering in 1997 from the University of Illinois at Urbana-Champaign (UIUC) and a M.S. in Electrical and Computer Engineering in 1994 from the University of Pennsylvania (UPENN). He worked for machine vision, government contracting, and research and educational institutions before joining the National Institute of Standards and Technology (NIST) in 2011. At NIST, he has been leading a project focusing on the application of computational science in biological metrology, and specifically stem cell characterization at very large scales. Peter’s area of research is large-scale image-based analyses and syntheses using mathematical, statistical and computational models while leveraging computer science foundations for image processing, machine learning, computer vision, and pattern recognition. He has co-authored more than more than 32 journal papers and eight books or book chapters, and close to 100 conference papers.
Nathan Hotaling is a Lead Data Scientist within the Information Resources Technology Branch at NCATS where he is responsible for overseeing and developing the next generation of artificially intelligent image analysis tools. He received his PhD in Biomedical Engineering and a masters in clinical research in 2013 from the Georgia Institute of Technology and Emory University. After his PhD, Nathan did post-doctoral research in a joint project between the National Institute of Standards and Technology (NIST) and the National Eye Institute (NEI) where he developed a platform to use in an Investigational New Drug application to the FDA for a therapy of Age-related Macular Degeneration (AMD) using induced pluripotent stem cells derived from patients with AMD. While pursuing this project he began to develop a platform to analyze high content image data-sets collected for drug screening and cell bio-manufacturing. This work led to his transition to his current position where he oversees the development of a scalable image analysis platform to non-invasively assess cell and tissue architecture, functionality, phenotype, consistency, and viability. Using this platform with novel machine learning and deep learning techniques he intends to unlock the next “omics” of cell analysis, Vis-omics, for both research and clinical projects.